concurrent (noun): Archaic. a rival or competitor.
โ dictionary.com
Two lines that do not intersect are called parallel lines.
โ Wikipedia
In this piece, I disagree with Joe Armstrong and Rob Pike, basing my argument on the differences between vending machines and
gift boxes (illustrated with drawings carefully prepared in Microsoft Paint).
Parallelism and concurrency are both very fashionable notions. Lots of languages and tools are advertised as good at these
things โ often at both things.
I believe that concurrency and parallelism call for very different tools, and each tool can be really good at either one or
the other. To oversimplify:
It's possible for both kinds of tools to co-exist in a single language or system. For example, Haskell appears to have good
tools for both concurrency and parallelism. But it's still two different sets of tools, and the Haskell wiki explains that you shouldn't use the concurrency tools if you're after
parallelism:
Rule of thumb: use Pure Parallelism if you can, Concurrency otherwise.
The people behind Haskell realize that one tool for both isn't good enough. It's a similar story with the new language
ParaSail โ it offers both kinds of tools, and advises
to avoid its concurrency features in parallel, non-concurrent programs.
This is in stark contrast to the opinion of some people primarily interested in concurrency, who think that their tools are a
great fit for parallelism. Rob Pike says Go makes parallelism easy
because it's a concurrent language:
Concurrency makes parallelism (and scaling and everything else) easy.
Likewise, Joe Armstrong claims that Erlang is great for parallelism because it's a concurrent language. He goes as far as to
say that seeking to parallelize "legacy" code written in non-concurrent languages is "solving the wrong problem".
What causes this difference in opinions? Why do Haskell and ParaSail people think you need separate tools for concurrency and
parallelism, while Go and Erlang people think that concurrent languages handle parallelism just fine?
I think people reach these different conclusions because they work on different problems, and develop a different perception
of the basic distinction between concurrency and parallelism. Joe Armstrong has drawn a picture to explain how he sees that
distinction. I'll draw you a different picture โ but first, here's a reproduction of his:
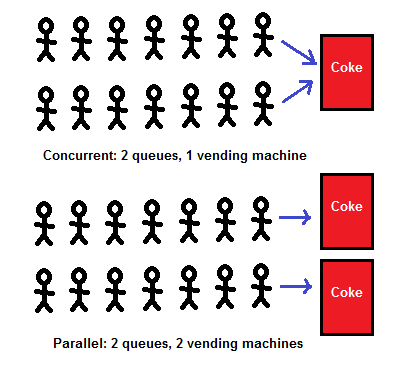
Actually many aspects of concurrency are present with just one queue, but I reproduced the 2 queues from Armstrong's original
drawing. So what does this picture say?
- "Parallel" means that you serve Coke faster.
- "Parallel" doesn't mean much else โ either way, it's the same concurrent problem.
- Who gets Coke first depends on who gets in line first.
- In a way it doesn't matter who gets Coke first โ they all get a can of Coke one way or another.
- ...except perhaps for the few last ones, if the machine runs out of Coke โ but that's life, man. Somebody has to be the
last.
Indeed, that's all there is to it with a vending machine. What about giving gifts to a bunch of kids? Is there a difference
between coke cans and presents?
In fact there is. The vending machine is an event handling system: people come at unpredictable moments, and
find an unpredictable amount of cans in the machine. The gift boxes is a computational system: you know the
kids, you know what you bought, and you decide who gets what and how.
In the gift boxes scenario, "concurrent vs parallel" looks very different:
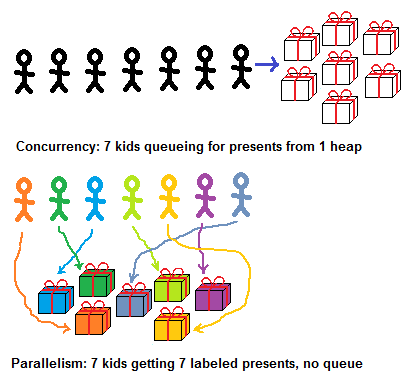
How is "concurrent" different from "parallel" in this example?
- Concurrent present-dealing is a lot like a vending machine: who gets what depends on who got there first.
- Parallel present-dealing is not like that: each present is labeled, so you know who gets what.
- In the concurrent case, a queue is necessary โ that's how you avoid two kids fighting over a present/two tasks corrupting a
shared data structure.
- In the parallel case, a queue is not necessary. No matter who gets where first, they all end up with the right
present.
Queueing in front of a gift heap is concurrent in that archaic sense of "rivalry, competition": you want to be there
first, so that it's you who gets the Lego set. In Russian, "concurrent" (pronounced kon-koo-rent) is the contemporary word for
"competitor".
With labeled gifts, there's no competition. Logically, labels "connect" each kid with his gift, and these are
parallel, non-intersecting, non-conflicting lines. (Why did I draw the arrows so that they do intersect? We'll
get back to it later; a good way to think of it is, kids'/processors' paths do cross as they search for their gifts/data โ but
those intersections are not conflicts over who gets what.)
Computation vs event handling
With event handling systems such as vending machines, telephony, web servers and banks, concurrency is inherent to
the problem โ you must resolve inevitable conflicts between unpredictable requests. Parallelism is a part of the
solution - it speeds things up, but the root of the problem is concurrency.
With computational systems such as gift boxes, graphics, computer vision and scientific computing, concurrency is
not a part of the problem โ you compute an output from inputs known in advance, without any external events.
Parallelism is where the problems start โ it speeds things up, but it can introduce bugs.
Let's proceed to discuss the differences between computational systems and event handling systems. We'll start with obvious
things, like determinism, but there are many more subtle consequences.
Determinism: desirable vs impossible
In computational systems, determinism is desirable because it makes life easier in many ways. For example, it's nice to test
optimizations and refactorings by observing that results didn't change โ which you can only do with deterministic programs.
Determinism is often not strictly required โ you may genuinely not care which 100 images you find as long as they all have
kittens in them, or exactly what approximation of PI you compute as long as it's somewhere between 3 and 4. Determinism is
merely very nice โ and possible.
In event handling systems, however, determinism is impossible, in the sense that it is a requirement for different
orders of events to produce different results. If I got there first, I should get the last Coke can, the last dollar in the
shared bank account, etc. If you beat me to it by a millisecond, then it should go to you.
Of course, for basic debugging, you can always run the system completely serially, handling one event a time. But once you
make it a bit more realistic and start handling events without first finishing everything that you were doing, you can no longer
expect a specific result.
Even in a debugging environment, if you replay an event log with two users racing to withdraw money from a shared bank
account, you'll run the thing twice and it might reach two different final states. The number of possible final states is
exponential in the number of conflicts.
Ouch.
Sign of parallel safety: determinism vs correctness
How do you know that you have no bugs due to the different ordering of events?
In computational systems, if you get the same result every time, you probably have no parallelism bugs โ even if the
result is busted. Dog pictures instead of cats means you have bugs โ but not parallelism bugs if it's the same dogs every
time.
In event handling systems, the only sure sign of not having parallelism bugs is if you always get correct
results.
With two users racing to withdraw money, you can't expect to always reach the same result. What can you expect, assuming the
bank isn't buggy? Many things. You (presumably) can never have a negative account balance, you (presumably) can't drain a bank
account twice, in effect creating money, etc.
If none of these wrong things ever happen, then you got yourself a functioning bank, otherwise you have a bug. With a
telephony system, it's similar โ except that there's a different set of requirements defining "correct results".
There's no general way to tell if there are timing-related bugs without understanding the aspects of the system
having nothing to do with timing.
Ouch!
Parallelism bugs: easy to pinpoint vs impossible to
define
With labeled gift boxes, parallelism bugs are trivial to spot โ even if the labels are in Chinese, and you can't read
Chinese:
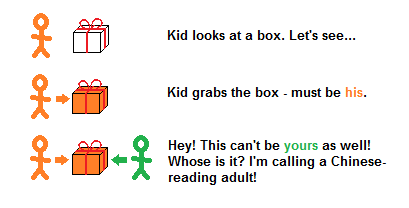
Even if you can't read the labels, knowing that they exist is enough. If two kids fight over a box, then something
is wrong and you call an adult who can read the labels.
If you're an automated debugging tool, and you don't know which task is supposed to process which data, it's enough to know
that a task shouldn't access data modified by another task. If that happens, you tell the programmer, so that
he properly assigns data to tasks without conflicts.
Such tools aren't hypothetical โ they're out there. Cilk comes with a tool that does that. Checkedthreads has a
Valgrind-based tool that does that.
Parallel Haskell doesn't have to do it โ because you have no side effects to begin with, guaranteeing zero conflicts when
things are evaluated in parallel. But even with dynamic checking instead of static guarantees, your parallelism bugs are
basically just gone.
The upshot is that in computational systems, you don't need to know much to flag bugs and to point to the exact
problem they cause. "Here's the box they were fighting over".
In event handling systems, a responsible adult must be much more knowledgeable to maintain discipline.
To be sure, there still is a weaker, but universal rule which always applies: you can't touch anything if someone is
already busy with it. You must wait your turn in a queue. An adult can make sure this rule is followed, which is better
than nothing. But it's not enough to prevent many common kinds of mischief:
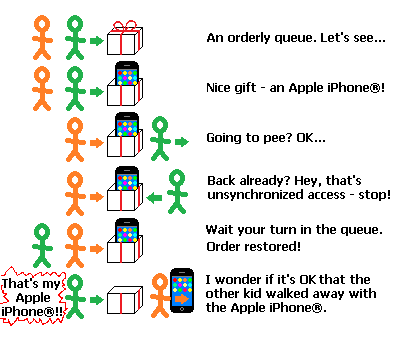
Someone approaching the presents without queuing is clearly wrong โ bug detected, order restored.
But someone unpacking a present, leaving the queue, and coming back to find that the present was taken by someone else could
be wrong โ or it could be OK. After all, that other kid waited his turn, so the only universal rule of event handling systems
was followed โ but not necessarily the specific rules of how we give out presents around here.
Here's how these problems look in code. The following buggy money transfer implementation can be flagged as buggy by an
automated debugging tool:
src.balance -= amount
dst.balance += amount
Here we have no synchronization at all โ src.balance can be modified by two processes without one of them waiting for the
other to be done with it. So you could lose some of the decrements or what-not. A data race detector like
Helgrind will spot this bug by monitoring memory accesses and observing the lack of synchronization โ just as easily as Cilk's
or checkedthreads' checker.
However, the version below is probably still buggy, but it would look fine to data race detectors:
atomic { src.balance -= amount }
atomic { dst.balance += amount }
Here, "atomic" means that everyone has to wait before modifying the balances in some sort of queue โ possibly a very fancy
sort of queue, but a queue nonetheless; more on that later. Orderly queuing makes data race detectors happy โ but is there still
a bug?
A thread could be suspended after decrementing src.balance but before incrementing dst.balance, exposing an intermediate
state where money is "temporarily lost". Is this a problem? Perhaps. Do you understand banking? I don't, not really โ and
Helgrind surely doesn't.
Here's a more obviously wrong program:
if src.balance - amount > 0:
atomic { src.balance -= amount }
atomic { dst.balance += amount }
Here, a thread could test that src.balance has enough money to withdraw a given amount, and go to pee. Another thread
arrives, makes a similar test and gets busy withdrawing the money. The first thread comes back, is put into some queue, waits
his turn and withdraws its own amount โ which could have become illegitimate, the first thread having withdrawn too much
already.
This is a lot like coming back and realizing that another kid has walked away with your Apple iPhoneยฎ. It's
a race condition which isn't a data race โ that is, it happens despite everyone's civil and polite
queuing.
What's a race condition? It depends on the application. I'm sure the last snippet is buggy, but I'm less
sure about the previous snippet, and it all depends on how the bank works. And if we can't even define "race conditions" without
knowing what the program is trying to do, we can't hope to automatically detect them.
Of course you can also not have race conditions. All you have to do is implement the entire withdrawal atomically,
and of course all approaches to concurrency let you do it.
The trouble with race conditions though, as opposed to, say, null pointer exceptions, is that you can feed the program
bug-triggering inputs again and again, and the thing will only reproduce once in a blue moon. Here's where deterministic,
automated debugging could be really handy, flagging the bug every time you feed those inputs. Unfortunately, it's impossible
with event handling systems.
"Ouch" again.
Queues: implementation detail vs part of the interface
With labeled gifts, you don't need a queue. Everyone can just go and take their present.
Or maybe not, not really. Thousands of kids getting thousands of labeled presents will need a queue or several, or else
they'd be running into each other. How those queues work doesn't matter in the sense that everyone ends up with the same present
anyway. The choice of a queuing method does affect how much time you wait for your present though.
That's why I drew the "logically parallel" lines connecting everyone to their labeled gifts so that they intersect โ even
though these aren't "actual conflicts" over who gets what. (I'm very careful with my metaphors and my Microsoft Paint art โ very
careful.)
4 processors accessing unrelated data items through a single memory bus is when "logically parallel lines technically cross",
and in effect processors will have to wait in some hardware-level queue to make it work. 1000 logically independent tasks
distributed to these 4 processors through a load balancing scheduler will again need queues to work.
There are almost always many queues even in a fully parallel, conflict-free system โ but they're an implementation detail and
they shouldn't affect results. You get the same thing no matter what your place was in any of the queues:
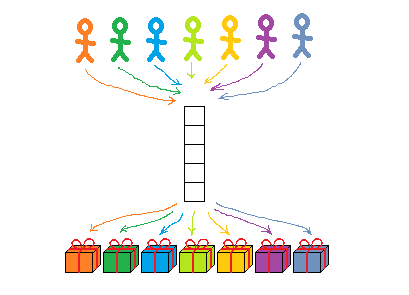
Conversely, in a concurrent system, the queue is right there in the interface:
- A semaphore has a queue of threads waiting to lock it, and who gets there first will affect results.
- An Erlang process has a queue of messages, and who sends a message first will affect results.
- A goroutine listens to a channel, and the order of writes to a channel affects results.
- With transactional memory, everyone failing to commit a transaction is queuing.
- With lock-free containers, everyone failing to update the container is queuing.
With event systems, you can have simple queues or fancy queues, but they are all explicit queues in the sense that order
often affects results, and that's OK โ or it could be a race condition, go figure.
With computational, parallel, conflict-free systems, order shouldn't affect results โ and you want tools to make sure the
system is indeed conflict-free.
Rob Pike shows in his slides how easy it is to build a load balancer in Go. Sure it's easy. Go is for concurrency and
concurrency is all about queues, and so is load balancing. Not that load balancers are very hard in any language, but yeah it's
particularly easy in concurrent languages.
But that's one part of the parallelism story; the next thing you want is either static guarantees of not having conflicts, or
dynamic checking. I'm not saying it can't be done in Go โ sure it can be done โ just that without having it, you underserve
computational systems. And once you do have it, it's a different set of interfaces and tools from channels and goroutines โ even
if underneath it's implemented with channels and goroutines.
Importance of preemption
So conflict prevention/detection is something computational systems need that concurrency tools don't provide. Are there
things that concurrency tools do provide that computational systems don't need?
Sure โ explicit queues, for starters. Not only aren't they needed, but explicit queues get in the way of computational
systems, because as we've seen, queues lead to race conditions that aren't data races and you can't pinpoint those.
Another thing computational systems don't really need is very cheap preemptable threads/processes.
With event handling systems, you sometimes want to react to many different events very quickly, which takes many preemptable
processes. You want to have 10000 processes stuck in the middle of something, and process number 10001 can still get activated
immediately when an event arrives. This can't work unless preemptable processes are very cheap.
With computational systems, it's nice to have cheap tasks that you can map to the relatively expensive OS threads โ
but tasks are not as powerful as processes. You don't activate tasks upon events. What tasks do is they wait in various
queues, and get processed when a worker thread becomes idle to run them. Unlike the case with goroutines or the like, you can't
have more tasks in flight than you have OS threads โ and you don't need to.
Tasks can be done nicely with fairly traditional runtimes โ as opposed to full-blown cheap processes/goroutines/whatever,
which seem to me to need more work at the low-level runtime system side of things.
This shows how platforms aiming at concurrency not only under-serve computational systems, but over-serve them as well.
(To be fair, it is theoretically conceivable to gain something from preemption in a computational system โ namely, it could
improve throughput if it lets freshly created tasks which are a part of the critical path preempt in-flight tasks which are not.
However, in my long and sad experience, a scheduler actually knowing what the critical paths are is little more than a
theoretical possibility. And a dumb greedy scheduler has no use for preemption.)
Tools aimed at concurrent event systems are not all alike, nor are tools aimed at parallel computational systems. They're
from the same family, but there are substantial differences.
- Erlang will not let processes share memory at all. This means you never have data races, which doesn't
particularly impress me because as we've seen, data races are easy to find automatically โ and race conditions are not at all
eliminated by not sharing memory. The nice thing though is you can seamlessly scale to multiple boxes, not just multiple cores
in the same chip.
- Rust won't let you share memory unless it's immutable. No easy multi-box scaling, but better performance on
a single box, and no need for data race detectors, which can have false negatives because of poor test coverage. (Actually it's
not quite like that โ here's a correction, also explaining that there
are plans to add parallelism tools to Rust in addition to its existing concurrency facilities.)
- Go will let you share everything, which gives the most performance at the price of what I think is a
tolerable verification burden. For data races, Go has a data race
detector, and race conditions will just happen in event systems anyway.
- STM Haskell will let you share immutable data freely, and mutable data if you explicitly ask for it. It
also provides a transactional memory interface, which I think is a cool thing to have and sometimes quite a pain to emulate with
other methods. Haskell has other concurrency tools as well โ there are channels, and if
you want to have Erlang's scalability to multiple machines, apparently Cloud Haskell does the trick.
Of course the other big difference is that you'd be programming in Erlang, Rust, Go and Haskell, respectively. And now to
computational systems:
- Parallel Haskell will only parallelize pure code. A static guarantee of no parallelism bugs at the cost of
having no side effects.
- ParaSail allows side effects, but disallows many other things, such as pointers, and as a result it will
only evaluate things in parallel if they don't share mutable data (for example, you can process two array slices in parallel if
the compiler is convinced that they don't overlap). Similarly to Haskell, ParaSail has some concurrency support โ namely
"concurrent objects" which indeed can be shared and mutable โ and documentation stresses the benefits of not using
concurrency tools when all you need is parallelism.
- Flow appears to be based on a pure functional core, which is restricted even further to let the compiler
fully comprehend the data flow in the program, and allowing it to target platforms as diverse as Hadoop and CUDA. Things
syntactically looking like side effects, parallel reduction, etc. are supposed to be a layer of sugar atop this core. At least
that's my impression from reading the Manifesto, which admittedly I
didn't fully understand ("if a morphism is surjective and injective, then it is a bijection and therefore it is invertible" is
more obvious to some of us than others.)
- Cilk is C with keywords for parallel loops and function calls. It will let you share mutable data and shoot
yourself in the foot, but it comes with tools that
deterministically find those bugs, if they could ever happen on your test inputs. What makes uninhibited shared mutable data
very useful is when you don't shoot yourself in the feet โ when a parallel loop computes stuff with whatever task-local
side-effect-based optimizations, and then the loop ends and now everyone can use the stuff.
- checkedthreads is a lot like Cilk; it doesn't rely on language extensions, and all of it is free and open โ
not just the interface and the runtime but the bug-finding tools as well.
I wrote checkedthreads, so this is the advertisement part; checkedthreads is portable, free, safe and available today in the very mainstream languages C and C++, unlike many
systems requiring new languages or language extensions.
With Cilk, there's an effort to standardize it in C++1y or some such, but Cilk wants to add keywords and the C++ people don't
like adding keywords. Cilk is available in branches of gcc and LLVM, but it won't run on all platforms at the moment (it extends
the ABI) and it hasn't been merged into the mainline for a while. Some newer Cilk features are patented. Not all of it is freely
available, etc. etc.
Cilk's big advantage, however, is that it's backed up by Intel, whereas checkedthreads is backed up by yours truly. And if
Cilk suits you and you decide to use it as a result of my checkedthreads-related blogging, I will have achieved my goal of
automated debugging of parallel programs getting some much-deserved attention.
The upshot is that not all concurrency tools are alike, and different parallelism tools are likewise different โ I don't even
claim to have pointed out the most important differences between my examples; it's hairy stuff. Still, they're two different
families, and the first thing to do is to pick the right family.
Conclusion
We've discussed differences between parallel, computational systems and concurrent, event handling systems. Areas of
differences include:
- Determinism: desirable vs impossible
- Sign of parallel safety: same results vs correct results
- Parallelism bugs: easy to pinpoint vs impossible to define
- Queues: implementation detail vs part of the interface
- Preemption: nearly useless vs nearly essential
For event handling systems, concurrency is their essence and parallelism is a part of some solutions โ typically
good ones (two vending machines is better than one). For computational systems, parallelism is their essence and
concurrency is a part of some solutions โ typically bad ones (a heap of gifts is usually worse than labeled gifts).
I hope to have gained more clarity than confusion by occasionally conflating "parallelism/concurrency" with
"computation/event handling". I also hope I didn't paint too dark a picture of event handling systems โ perhaps there are
automated verification strategies that I'm not aware of. However it came out, I don't claim that my viewpoint and terminology
usage is "the" way of looking at this.
There's value in the angle preferred by some people interested in event handling systems โ "concurrency is dealing with
several things at a time, parallelism is doing several things at a time". From this angle, parallelism is an implementation
detail, while concurrency is in the structure of the program.
I believe there's value in my perspective as well โ namely, "concurrency is dealing with inevitable timing-related
conflicts, parallelism is avoiding unnecessary conflicts" โ "vending machines vs labeled gifts". Here's how the two
look like โ now with parallel arrows disentangled, as they logically are:
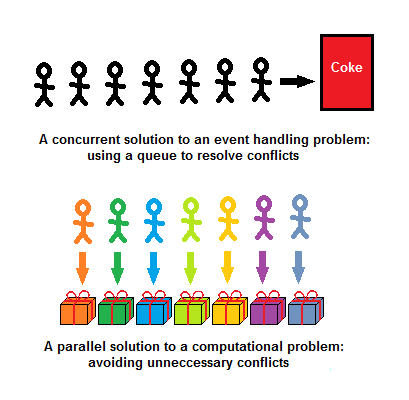
The most important takeaway is that computational code, as opposed to event handling code, can be made virtually bug-free
rather easily, using either automated debugging tools or static guarantees.
Handling parallelism using its own tools is nothing new. Rob Pike's work on Sawzall, a specialized parallel language where
code is always free from parallelism bugs, predates his work on the concurrent language Go.
However, tools for concurrency appear "louder" than tools for parallelism at the moment โ and they can handle
parallelism, albeit relatively badly. Loud and bad often crowds out the less visible better. It'll be a pity if better support
for parallelism won't be developed as a side effect of "loud concurrency" โ or if such support atrophies where already
available.
I'd go as far as replying to Armstrong's "parallelizing serial code is solving the wrong problem" with "using 'bare'
concurrency tools for computational code is applying your solution to the wrong problem". The simple fact is that C parallelized
with the help of the right tools is faster and safer than Erlang.
So here's to "using the right tool for the job", and not having anyone walk away with your Apple iPhoneยฎ.
I'd say your perspective is even better than "using the right tool
for the job" โ it's actually identifying the job itself first!
Parallel solutions become easier if you realize that some patterns
can be applied in general, with well defined synchronization points โ
all of which can be automatically tested and *do not* imply race
conditions. Like implementing a fork-join API and then creating more
elaborate APIs on top of that. Like your own checkedthreads.
If you need to solve a concurrent problem, then race conditions are
domain-specific and you'll just have to spend a good time modelling a
solution, otherwise that can'o'worms will be popping open in no
time.
I'm a game developer. Games often need to optimize for concurrency
and parallelism in the same package. I've been finding it very useful to
be more knowledgeable about when and how to work on each of those sets
of problems, thanks to works like yours.
I really wonder how much can be done about race conditions in event
handling systems, perhaps not in a wholly domain-independent way but
still in a relatively broadly applicable way. I've never worked on very
big systems of this sort โ not on the kind where it's many developers
and you have to keep the system correct for years in the face of
continuous updates. The ability to hunt down bugs in computational
systems as I describe is something that took me years to figure out
through many steps; I wonder what I'd know if I worked enough time on
big enough event handling systems.
It really is a shame that engineering has become so rare in this
profession. Most "kids" these days are just using node.js, thinking it
is cutting edge and clueless as to the ramifications.
Similarly, Go is also a "hot new language" used by people who don't
understand what they are doing.
Further the attempts to divide between "concurrency" and
"parallelism" is just leaving people such as this author confused.
To provide scalable, robust systems, you need three things:
immutability, process separation (Eg: message passing) and this is the
most importantโ knowledge of when a process crashes.
Go provides only one of those three things. Go is a terrible language
for robust programming as a result.
But go has superior single machine performance, and since we have a
culture of people who think going really fast on a single CPU is super
important compared to being able to optimize across a cluster of
machinesโ go is popular. (The author falls into this same trap, when he
fails to appreciate erlang's multi-node scalability. It's not just a
line item.)
I can't speak to haskell and the other explicitly parallel
languages... but if you are writing code, and you're splitting work up
among multiple processes/threads, etc and you have no way of knowing
when one of them dies (in order to handle it) you're going to have
serious problems.
Anyone who chooses go is incompetent. (And yes, Rob Pike is
incompetent. This industry also suffers from the "works for famous
company, therefore shall not be questioned" fallacy.)
Finally, this article misses the point completely because it leaves
out the key issue of knowledge of process crashing. This tells me the
author hasn't spent enough time working on these kinds of systems, and
is instead spreading his opinion based on fashion.
Languages are not like some designer's fall line. You don't critique
them based on how you feel about them (and you obviously feel that
so-called (and misnamed) parallelization is supperior to concurrency,
which shows you're lacking an understanding of concurrency).
Which lets you dismiss erlang with the flippancy of someone who
decided he didn't like the way its syntax looked and so wasn't going to
be bothered to understand why someone would use it. (which may not be
the case, but your flippancy is like someone like that.)
Engineer
PS: Apparently "Yes" doesn't count as indicating one is human. Excuse
me for capitalizing.
"you obviously feel that so-called (and misnamed) parallelization is
supperior to concurrency, which shows you're lacking an understanding of
concurrency"
Erm... I never said one was "superior" to the other, in the same way
that spoons aren't superior to knives; I just said you can hurt yourself
more badly with knives, and you can't eat soup nearly as
conveniently.
"To provide scalable, robust systems, you need three things:
immutability, process separation (Eg: message passing) and this is the
most importantโ knowledge of when a process crashes."
In the general case, this is bunk, and I hope that people
comprehending what they read will walk away knowing exactly why. Which
is not to say that these properties give you no value under any specific
circumstances.
>I don't know if Go has data race detectors
Go now has a builtin data race detector:
http://golang.org/doc/articles/race_detector.html
Thanks โ fixed the article. I wonder how easy it is to build
something like the Cilk's deterministic checker for fork/join code on
top of this.
Concurrent is synonymous with simultaneous and is always correct
terminology when describing something that either exists at the same
time and/or is happening at the same time. Parallel, on the other hand,
really refers to something physical, e.g. physical paths or layout in
hardware, although it has become common (acceptable?) to use the term
parallel in place of concurrent.
In Armstrong's second example replacing the word Parallel with the
word Concurrent is just as correct/proper. In example one, the queues
are concurrent and in parallel and the processing is
pseudo-concurrent.
An example, say Ted is in Redmond and Bill is in New York, it's 10
AM, and both are working on the halting problem. It's correct to say
they're working on the problem concurrently, or in parallel. But if Ted
and Bill were sitting next to each other it would be a concurrent effort
laid out in parallel.
Yossi, when working with systems with a lot of events I've found the
Reactive Programming approach very productive and less error-prone than
the generic one. I'll describe what I mean by that as different people
mean different things when talking about RP / FRP.
Basically instead of events you model your application as state cells
and rules that react to some cells changing and change some other cells
in response triggering a cascade of (implicit) events. First write to a
cell starts a transaction and it ends when there are no more rules
pending. If some rule gets triggered twice per transaction it's either a
circular dependency and a bug (and the error can be generated pointing
directly at the culprit) or it's the wrong order for the rules to run,
so they get adjusted, transaction gets rolled back partially and the
rules are rerun.
Such a system provides some additional guarantees, catches a number
of bugs that are horrible to debug in other approaches, data
dependencies are tracked in such a way that it reduces both code
duplication and errors etc.
Also if certain conditions trigger a bug, state changes are atomic,
so the system is left in a valid state after the error and it can safely
move on to handle the next request.
I didn't cover concurrency in such a system but there are good
properties for that as well.
Note that such a system has no explicit events or dependency graph โ
both are implicit.
Here are some links
https://bitbucket.org/mlk/reaction โ my old library
for this kind of thing
https://pypi.python.org/pypi/Trellis โ the original
library by PJE I've derived Reaction from
https://maluke.com/old/txns โ an old intro to this
topic (that I wrote), might help flesh out what I was writing
above
https://plus.google.com/110081677554331361663/posts/58fBBnEYnrG
โ another intro, also by yours truly, written more recently
https://plus.google.com/110081677554331361663/posts โ
more posts on the topic (some basic implementations and JS demos
included)
I understand that this is not universally applicable, but still I
think it's something a lot of people would benefit from learning
about.
Do you have any impressions of the quality of automated debugging
tools are in languages aimed at distributed-memory parallelism in HPC?
I.e., things like Cray's Chapel, Co-Array Fortran or Berkeley's Unified
Parallel C.
("Manual" distributed memory parallelism, i.e. MPI, is probably yet
another can of worms...)
@Adam: I'm an embedded type, not an HPC type, at least currently and
most of the time (I was involved in parallelizing stuff at process
granularity over the CPUs of a compute farm recently, but those weren't
very big projects.) So I wouldn't know; I do think that MPI might be
pushing one into this corner of, it lets you do true concurrency, you're
probably using it for parallelism, and now you're stuck without good
automated debugging tools (are you?.. it just looks like one of those
things without such tools but I may be just ignorant.)
@Sergey: interesting; I wonder how this works for, say, the shared
bank account case. (Maybe a very basic question โ I just never looked
very deeply into RP, since I don't deal with event handling a whole
lot.)
For simple cases when there's just as single piece of state/data to
be synchronized it effectively works as either regular optimistic or
pessimistic locking (depending on the implementation).
The benefits are really only seen when event handlers trigger more
events, especially when there are multiple handlers per event (which is
very common in UI for example, because the same data is usually
displayed in multiple forms at the same time).
The thing is that normally each triggered event would queue up the
handlers that's where the concurrency bugs creep in. With RP, if the
events (and their handlers / callbacks) are related, that is tracked and
incorrect behavior is detected as it happens. In other words there are
constraints on the rules defined in the system and if the rules abide by
those constraints, then when you add more and more of them the system as
a whole is guaranteed to either work correctly or to raise exceptions
the very moment rules conflict (because each rule can be valid on it's
own, but conflict with another valid one).
>I wonder how easy it is to build something like the Cilk's
deterministic checker for fork/join code on top of this.
It's impossible... or it's already the deterministic checker if you
wish.
Cilk's checker is very limited, it requires structured nested
parallelism (enforced by Cilk model, not enforced by Go) and absence of
mutex or any other shared memory communication (not enforced by
both).
So if you use structured nested parallelism and do not use shared memory
and use deterministic reduction order, then it's the deterministic
checker. Typical Go programs do not satisfy that requirements
(inherently non-deterministic).
not sure about this, seems to me like the concurrency constructs can
be used by a compiler to set up parallelisation, and do it flexibly,
with the programmer learning not to do things that prevent this (late
optimisation). Also currently can't see much of a win from use on
serial-centric CPU hardware, so maybe we really need to wait until
consumer parallel hardware (GPU, APU, Cloud) is settled enough, and use
cases are clear enough, for the effort to be put in on compilers.
in terms of bugs, aren't individual race conditions going to be
generally limited to a unit, so these problems don't get that much worse
with increasing program complexity, and so aren't so much of a
high-level 'structural' issue.
what about Clojure? Quasar?
http://blog.paralleluniverse.co/post/49445260575/quasar-pulsar
I think you're missing the point regarding Haskell's concurrency vs.
parallelism.
"Rule of thumb: use Pure Parallelism if you can, Concurrency
otherwise."
This isn't talking about parallelism vs. concurrency, the concepts,
this is talking about Parallelism vs. Concurrency, the modules
(capitalized). The emphasis here is on using PURE when you can (meaning
no side effects like disk IO).
In Haskell, when you're working with pure computations, Haskell can
make guarantees about what's going on and effectively manage the
concurrency for you. From this concurrency, you can get parallelism
almost for free (meaning no extra programming restructuring on the devs
part). So the module is called Control.Parallel.
If you want to be doing parallel IO with Haskell, concurrency can't
be managed automatically for the dev. This is Control.Concurrent.
"The people behind Haskell realize that one tool for both isn't good
enough."
Both Control.Concurrent and Control.Parallel are for parallelism! The
difference here is whether you're parallelising pure or side-effectful
computations and by extension can get free concurrency. Haskell doesn't
have different tools for parallelism and concurrency. It has different
tools for parallelising pure vs. effects.
If you have parallelism, you have concurrency. You can have
concurrent processes without parallelising them though.
Yossi you should check out Akka
http://akka.io
I could be wrong, but I believe it's a tool for both concurrency and
parallelism
@Dmitry: I think that even if you limit the Go program in question to
strict fork/join parallelism, it's not clear you'll get deterministic
bug-finding โ for instance, the tool might not notice write-after-read
conflicts (it's easier to notice read-after-write) and then the question
is if there's a scheduler which changes the order of things so that
write-after-read becomes read-after-write, or is it handled in some
other way. There are also questions such as what happens if you cancel a
task, is any sort of sharing supported after all as is the case with
Cilk's patented "hyperobjects", etc.
@Clojure and Scala guys โ if you want to start a conversation, then
go ahead and start it :) Please explain what these things you link to do
in the shared bank account case and the case where two tasks attempt to
modify the same element of a shared array, if such scenarios are
expressible to begin with.
Engineer, wow you made me laugh.
If you are going to spend time on parallelizing code for better
performance the first step would be to ditch erlang completely and move
to C or C++.
As for go it's "good for what it is" I guess. Same applies here but
the makers are not operating under the same kind of delusion.
> With lock-free containers, everyone failing to update the
container is queuing.
That's not the case with ready-only containers, where "update" happens
via creating a new copy.
The simplest example of such containers are "copy-on-write" collections
from java.util. A more sophisticated examples are Clojure data
structures, where creating a copy involves structural sharing, which
makes this approach much more efficient.
@sesm: so what happens if the container is a list of users and I come
to register as BillG and someone else tries to do so as well? Who wins?
Isn't there queueing involved in that case?
@Yossi Kreinin
There are two dimensions in your question:
1. Appending to immutable list.
2. Using a shared variable to store users.
Regarding 1: Suppose we had list (a b c) in the beginning. When we
append BillG to list, we will get a new list (BillG a b c) as a result.
There will be structural sharing involved: the tail of the new list will
be the old list. However, the original list will stay untouched, anyone
performing computations on it will not be affected.
If one thread append BillG, and the other thread appends YossiK at the
same time, the first will get (BillG a b c) and the second will get
(YossiK a b c), and it's not important, which one was executed
first.
Regarding 2: If we have a shared list of users and we want to add
users from multiple threads, we need coordination. In the Clojure land
the right coordination mechanism would be agents (http://clojure.org/agents) , which, not surprisingly,
have a queue inside them.
Notice, however, that the need to use a queue comes from the problem
definition, but not from the containers themselves. When you don't need
coordinated updates of shared state, e.g. you just want to read or use
some modified version in thread-local computations, there is no need to
queue.
(BTW, I'm new to Clojure and feel awkward speaking for it. The
clojure.org website says it all much better)
Good information.. You have provided good information. even you have
provided examples.. Those examples are nice and best.. Thanks for the
posting this article.!
Scala also has different tools for different purposes.
On one hand, there's the "parallel collections" feature which can be
used to exploit data parallelism easily. However, there's no tool that
would check if the executed operation really is pure. There were talks
about having purity checked statically through the type-system but
nothing like that has entered Scala mainstream yet.
On the other hand, there's akka whose building blocks are actors
similar to erlang so similar conclusions would apply.
To answer your question how you the banking transaction could be
build I think it makes sense to further divide your classification of
concurrency.
Lots of concurrency problems can be solved by not allowing any shared
mutable state at all. Those can be very well represented by
actors.
The banking transaction problem can only be solved this way if the
complete world state would be represented by one actor which would
effectively mean serializing all operations and giving up concurrency
completely.
So, thereโs another class of concurrency problems where the amount of
data to is too much to completely serialize all accesses to it. For
those situations akka has the concept of โTransactorsโ to coordinate
atomic operations across actors. [1]
It seems you are arguing about two different questions in your
post:
1. Should concurrency tools be used to implement parallelism?
2. What are the tools to solve the hard concurrency problems?
Regarding the first I would agree that there probably should be
specific tools for that. However, parallelism is arguably simpler to
handle than concurrency. A parallelism tool should a) help to make sure
that the operation actually is a โcomputational systemsโ and b) solve
the concurrent problem of how to schedule a number of parallel tasks
onto a smaller number of CPUs.
Regarding the second question, Iโd say that concurrent problems often
already come with established protocols about how to solve the race
conditions (like in banking). A tool should be evaluated by how easy it
is to represent those protocols in code.
[1] http://doc.akka.io/docs/akka/2.1.4/scala/transactors.html
I agree regarding (1); as to (2) โ I guess that's so, I just wonder
how generic these protocols are (or how many families of such protocols
exist) and how easy it is to make sure that you actually follow them. In
particular, it's technically easy to have bugs with actors โ it's easy
enough to not have bugs as well perhaps, but also to have them;
I wonder if there's anything except "code correct by design" with these
things.
You've an interesting perspective, and I appreciated reading
this.
That said, I think you mishandled the banking examples.
Banking needs to be reliable, and hardware can fail. So a high volume
banking system would use both what you call "concurrency" and what you
identify as "parallelism".
First, you have a concurrent externally facing system. This generates
a replayable log which is strictly ordered and which includes references
to some elements (e.g. accounts) which can be handled using parallelism.
(For example, you can add markers to the log, indicating time intervals,
and you can batch together requests arriving in the same time interval โ
clients need to wait for their time interval to be completed before they
get the results of their request.)
Once you have this log, you can play (or replay) it on multiple
machines and expect to get the same results.
I don't think I mishandled them as much as oversimplified them to
make a point.
I think that the following article by Robert Harper is quite
interesting:
http://existentialtype.wordpress.com/2011/03/17/parallelism-is-not-concurrency/
@Yossi, the "immutable" data structures of functional programming
languages is a bit of a lie.
There's always something mutable, and in this case it's the pointer
to the data structure. So conflicting updates will race to change the
pointer.
The benefit of that is, that everyone can keep a view of historical
checkpoints in the data structure with little cost, and this could be
beneficial for some purposes.
I don't know if I completely understood the argument;
here is how I read your article:
- systems where requests depend on each other or depend on a common
shared state introduce locks, these limit the degree of concurrency;
here one cannot realize the promise of parallel processing completely.
So you classify these as concurrent systems.
- scientific problems can better utilize parallel processing, because
usually they do not have dependencies on shared state; so you classify
them as real parallel problems.
Still: even with concurrent systems, if they run in multiple
preempted threads you can have some degree of parallel processing.
Concurrency can be done via cooperative multitasking; here you do not
have parallel processing, unless
you run multiple preemted threads where each preemted thread runs its
set of coopertively scheduled threads.
I am confused.
I didn't mean that you can't do parallel processing in concurrent
systems; I meant that concurrent systems are not deterministic while
parallel systems can be, and discussed the implications.
Thanks, that clears it up.
I think you're making too big a deal out of the indeterminacy of
queuing systems. Testing in general never gives guarantees; only proofs
can do that. And yet, after almost 60 years of computer programming,
somehow provably correct code has not caught on. Hmm.
Either we're all the world's biggest bullshit artists, or the
engineering tradeoffs of rapid development time and evolutionary
flexibility of designs are worth a few bugs here and there.
Go closes off a lot of the undefined behavior found in C and C++, but
leaves some concurrency related undefined behavior. I believe that this
will be an acceptable engineering tradeoff for the next few decades,
just like C and C++'s was for the last few decades.
Fork/join is nice, but how many problems really fit a pure fork/join
paradigm? Any time you need any kind of communication between tasks,
even for something as trivial as having them report back what percentage
done they are, you start needing a queuing/messaging system. And then
you are back to the Golang model.
I almost wonder if fork/join needs any language support at all. I
remember being "wowed" many years ago by the built-in support UNIX had
for fork/join. It's just as simple as this:
#!/usr/bin/env
thing1&
thing2&
thing3&
wait
Bash is often reviled as overly complex and baroque, but what could
be simpler than this? Another fun technique is to use a Makefile and
then use make -j 4 to run only 4 processes at any given time.
It might be worth exploring how something like checkedthreads could
be implemented in golang. I wonder if you would need a library at all,
or whether it would become more of a design pattern. My suspicion is
that it would be the latter...
People prefer having a bigger toolbox to a smaller, even if the big
toolbox has some sharp things in it. This is one reason why threads
exist at all, when we all "know" that processes are better. Threads
solve a superset of the problems processes solve (if we ignore hacks
like shared memory that make processes more like threads). I think
Golang will win out.
If you're speeding up computations, then a whole lot of things can be
done using pure fork/join code; you'll be hard-pressed to find things
that can't be, and those things are often still quite close to pure
fork/join. Reporting progress as you mentioned is not a
computational problem; do you need a queue there? โ not really, you can
write to a shared variable and whoever shows progress to the user just
grabs some value from that variable, doesn't matter which value exactly.
No big deal.
Library vs design pattern โ you want a library to be able to have
checking tools. If you think you don't need such tools, perhaps you're
right โ it depends on the size of your team, the size of your system,
and the tolerance your customers have for bugs (mine have rather low
tolerance โ they're car vendors). Note that dynamic checkers, unlike
static verifiers, did catch on โ Valgrind is a prominent example,
language-level array bounds checking is another, and Go's own race
detector is perhaps the most fitting example, for someone who likes Go
but dislikes automatic bug finding tools.
As to Go "winning out" โ I didn't say otherwise, "and frankly, my
dear, I don't give a damn".
I never said I disliked static verifiers. I just happen to think that
the best place for static verification to happen is usually in the
compiler. That's why we have type systems, after all.
C and C++ have spawned a lot of static verifiers like Coverity,
cppcheck, and so forth, as well as compiler settings like -Wall,
-Wextra, and -Weffc++. Let's be honest... 99% of what these verifiers do
is point out that you are about to encounter boneheaded C++ behavior
#3445 (implicit narrowing), or #1424 (primitives stored on the stack are
uninitialized by default), and so forth. If you didn't have boneheaded
language behaviors, you wouldn't need these kind of checks.
The Linux kernel guys have done some interesting things with the
"sparse" tool which go beyond just fixing language messes. For example,
you can use sparse to annotate that code is running in interrupt
context.
As someone who builds middleware, I have a healthy fear of it. I
think if you can do something by just relying on what the OS gives you,
you should. It's kind of a shame that more people don't use
multi-process designs, since the OS already provides all the isolation
you need. Hopefully projects like Chrome and postgres will make
multi-process designs no longer taboo.
An interesting perspective on the terms "concurrency" and
"parallelism" can be gleaned from the book "Concepts, Techniques, and
Models of Computer Programming," which is a wonderful introductory text
on the semantics on programming languages.
In that text, "concurrency" refers to a property of language
semantics (as seen by the programmer) when it can express the concept of
multiple execution paths that progress at the same time. To aid in
reasoning, these paths can be considered to be part of a single sequence
of interleaved atomic segments of execution from the various paths. This
is because no matter how many cores the code runs on, the code's pattern
of access to the store will be observationally equivalent to some
interleaving of code segments.
On the other hand, "parallelism" is a detail of the implementation of
the execution of a program. It refers to the situation where paths in
the program are *actually* executing simultaneously on different
processing cores. In this definition, it is an optimization detail of
the implementation rather than part of the language semantics.
Interestingly, these two concepts are actually completely
independent. Superscalar CPUs regularly derive and take advantage of
opportunities to perform parallel execution of instructions from a
sequential program stream. Many programming languages are now being (or
already have been) developed that try to do the same thing; they
constrain the data models such that the compiler can automatically find
opportunities to run segments of a *sequential program* in parallel.
On the other hand, there are plenty of languages that have explicitly
concurrent semantics, but only interleave operations on a single
processor core.
Somewhere in-between, there are concurrent languages that execute
their concurrent language-level tasks in parallel on multiple cores or
even entirely different computers. Here we hit the issues of
determinism, and in general the ability to reason about the behavior and
performance of programs written in these languages.
The book discusses several different models of concurrent languages.
The first is declarative concurrency, which provides fully-deterministic
execution of a program composed of concurrent tasks. When the
implementation can schedule these tasks in parallel across multiple
cores, this allows the programmer get the deterministic behavior of a
sequential program along with more control over the parallelism than a
sequential program with automatic parallelism. Of course, concurrency
has other purposes besides allowing parallelism, and many of these apply
to declarative concurrency as well.
The book also presents some non-deterministic concurrent language
models such as message-passing concurrency and shared-state concurrency.
Message-passing concurrency allows you to introduce non-determinism
without creating the arbitrary program interleavings that occur by
default in shared-state concurrency, at the cost of some implementation
overhead. The main benefit of shared-state concurrency is, of course,
that it's very close to the underlying machine model and can be
implemented very efficiently.
So, to apply this taxonomy back to your original discussion, the
systems that are good at what you call parallelism have been optimized
for the purpose of accelerating mostly-sequential codes by running
chunks of processing simultaneously on multiple cores, while those you
mention as good at concurrency are optimized for nondeterministic
concurrency, i.e. choosing between multiple execution paths at
scheduling points based on runtime information, such as asynchronous
events.
The interesting thing is that you list Haskell in both camps; from
the point of view of the CTM book, it's clear why. Haskell is a
declarative language, and it is easier to analyze and parallelize the
behavior of a declarative program, though Haskell's laziness presents
some challenges. Extending a declarative core language with limited
concurrency primitives is also far easier than trying to reign-in the
arbitrary interleavings of a shared-state concurrency model.
I also think it's interesting that some of your 'good at paralellism'
systems are essentially tools for doing the difficult analysis problems
that shared-state concurrency problems create. This seems to be akin to
trying to constrain a subset of a shared-state program to a declarative
concurrency model and then providing some tools to find where you
accidentally violated the constrained model. I assume they are worth the
added trouble because they allow for a lot of flexibility and efficiency
in the hands of expert programmers over systems that are
declarative-by-default or which primarily do message-passing
concurrency.
Thanks for the interesting post!
Thanks for the interesting comment :)
Thanks for an elegant post. One thing that I think is missed is that
even "computational systems" generally benefit from cheap context
switches. In most applications, the "cheap tasks" that computational
systems deal with often need to wait for I/O of sorts, at which point
being able to switch to another task and be efficiently activated back
when the I/O operation finished improves both latency and
throughput.
Also, I would be curious about adding another dimension in the
comparison of tools designed for parallelism โ the level of granularity
that they encourage people to use for expressing the parallel parts of
their computation. What's the overhead (if any) paid for expressing the
fact that iterations of a loop can be run in parallel? Is it worth doing
for extremely short iterations? Is the program penalized (compared to an
equivalent sequential program) if it ends up running on a single
processing unit? Do I, as a programmer, need to tune this level based on
the parallel processing capabilities of the particular machine that my
program is running on, or am I encouraged to express all the parallelism
possible and expect the system to take care of exploiting it at the
right extent?
I'm intrigued but not convinced. Can somebody provide a couple of
examples, and show me why they're easier to make parallel in Haskell or
Sawzall than Go or Rust?
Go is a young language and Rust is even younger. But then no
comparison can ever really be fair.
I never said one language would have nicer-looking example code than
the other, only that automated debugging tools could be developed for
some frameworks but not others. Go for instance could easily grow a
framework for parallelism as easily debuggable as say Cilk, I'm just
saying it needs to do this or its parallel code will not be amenable to
automated debugging tools.
Examples showing that Go requires discipline even though it "has
concurrency baked in" are here:
https://blog.mozilla.org/services/2014/03/12/sane-concurrency-with-go/
Now for concurrency I don't suggest any improvements, but for
parallelism one improve the situation by coding against a framework
coming with automated debugging support as Cilk or checkedthreads in
C.
As to Rust โ the HN thread discussing this article has in its topmost
comment the opinion of a key person in Rust mostly agreeing with what I
say:
https://news.ycombinator.com/item?id=5711232
I agree to an extent but most of the problems are solvable as opposed
to going to C++. Getting to the level of C++ for hft is really not easy
in C++ world and fighting bug war on 'hot' C++ code requires steep
learning curve for even a good c++ programmer. Even some jvm writers
find hft c++ code not so easy. Objective C code is relatively straight
fwd. and you are not battling a great concurrency battles. Most mobile
programmers are writing high level apps not really knowing under the
hood stuff.
Concurrency: two queues, two vending machines, one selling pop and
one selling candy.
Parallelism: two queues, two identical vending machines.
Concurrent by definition means "at the same time" so there must be
multiple vending machines in the concurrent example.
Post a comment